Understanding Algorithmic Bias in AI
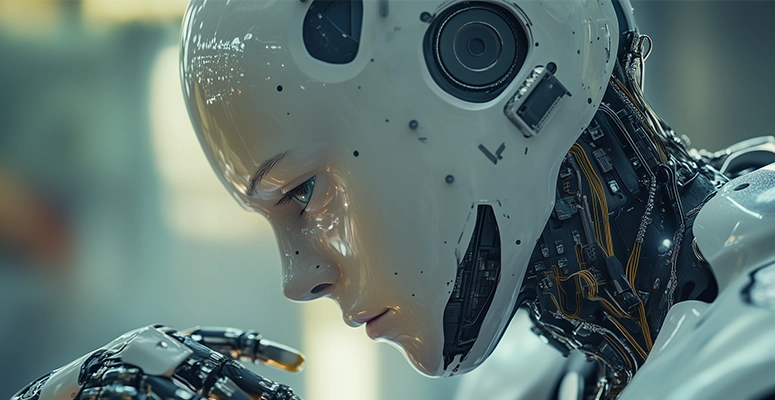
Algorithmic bias in artificial intelligence (AI) refers to systematic and repeatable errors in AI systems that create unfair outcomes, such as privileging one arbitrary group over another. Imagine applying for a job and being rejected not because of your qualifications but because the AI used by the company favors certain demographics over others. Addressing this bias is critical as AI increasingly influences various aspects of society, from hiring practices to law enforcement.
History and Evolution of Algorithmic Bias
The awareness of algorithmic bias dates back to the early days of automated systems. One of the earliest examples is the use of biased data in credit scoring systems which often resulted in minority groups being unfairly denied loans. As AI technologies advanced, these biases became more pronounced. For instance, in 2016, ProPublica published a groundbreaking study revealing that an algorithm used in the criminal justice system was twice as likely to falsely flag Black defendants as future criminals compared to white defendants. This increasing awareness has led to more scrutiny and calls for fairness in AI development.
Sources of Bias in AI
- Bias in Training Data: AI models learn from the data they are trained on. If this data is biased, the model will likely replicate these biases. For instance, a hiring algorithm trained on historical data that favors certain demographics will continue to favor those groups.
- Bias in Algorithm Design: The design of the algorithm itself can introduce bias. This includes the choice of features, the decision-making process, and the evaluation metrics used. For example, if an algorithm is designed to prioritize candidates with certain keywords that are more commonly used by one gender, it may inadvertently favor that gender.
- Human Oversight and Bias: Humans involved in the development and deployment of AI systems can unintentionally introduce their own biases, affecting the overall fairness of the AI system. This can occur through subjective decisions made during the data collection, algorithm design, or interpretation of the AI's results. For example, if developers predominantly belong to a particular demographic, their perspectives might inadvertently shape the AI in biased ways.
Impacts of Algorithmic Bias
Real-World Consequences
Algorithmic bias has significant real-world impacts, including:
- Social Inequities: Biased algorithms can reinforce and amplify existing social inequalities. For example, predictive policing algorithms may disproportionately target minority communities, leading to increased scrutiny and harsher enforcement. This was evident in the case of the "PredPol" software used in the U.S., which was found to disproportionately police neighborhoods predominantly populated by minority groups.
- Economic Disparities: Bias in financial algorithms, such as those used for loan approvals or credit scoring, can result in unequal access to financial resources, perpetuating economic disparities. For instance, a study found that algorithms used by some lenders were more likely to deny loans to applicants from minority groups, even when their financial profiles were similar to those of approved applicants from majority groups.
- Legal and Ethical Concerns: Biased AI systems raise numerous legal and ethical issues, including violations of anti-discrimination laws and ethical standards for fairness and justice. For example, the COMPAS algorithm used in the criminal justice system has been criticized for its racial bias, leading to ethical debates and legal challenges about its use in sentencing and parole decisions.
Mitigating Algorithmic Bias
Detection Methods
Statistical Techniques To detect bias, statistical techniques such as disparate impact analysis can be used. This involves comparing the outcomes of different demographic groups to see if there is a significant disparity. For instance, an algorithm that disproportionately selects male candidates over female candidates for job interviews can be flagged using statistical disparity measures. Another technique is the use of fairness metrics, which help in quantifying the degree of bias present in an AI system.
Bias Audits: Bias audits involve a thorough examination of AI systems by independent auditors. These audits can help identify and rectify biases that may not be apparent to the developers. For example, the AI Now Institute has recommended regular bias audits for all high-stakes AI systems. Regular audits are essential to ensure that AI systems remain fair over time as biases can emerge due to changes in data or context. An effective audit process includes reviewing the training data, testing the algorithm with diverse datasets, and assessing the decision-making process for any unfair patterns.
Mitigation Strategies
Inclusive Data Collection: One effective strategy for mitigating bias is to ensure that training data is diverse and representative of all relevant demographics. This helps prevent the model from learning biased patterns. For example, in facial recognition, using a diverse dataset with a balanced representation of skin tones and ethnicities can improve accuracy across all groups.
Fairness Techniques: Several algorithmic fairness techniques can be applied to reduce bias. These include re-weighting training data, modifying the learning algorithm to be less sensitive to biased patterns, and using fairness constraints during the optimization process. For instance, techniques like "Fairness Through Awareness" involve modifying algorithms to ensure that they do not discriminate based on sensitive attributes. Additionally, methods like "Adversarial Debiasing" train models to minimize bias by introducing adversarial networks that penalize biased predictions.
Ethical Frameworks and Guidelines
Principles of Ethical AI: Adopting principles of ethical AI is crucial for guiding the development and deployment of fair AI systems. Key principles include transparency, accountability, and inclusivity. These principles ensure that AI systems are designed and used in ways that promote fairness and justice. For instance, Google's AI Principles emphasize avoiding creating or reinforcing bias and being accountable to people.
Global Initiatives: Several global initiatives aim to establish guidelines for ethical AI. Organizations like the European Commission and the IEEE have developed frameworks to ensure that AI systems are developed responsibly. The European Commission's Ethics Guidelines for Trustworthy AI outline key requirements such as human agency and oversight, technical robustness, privacy and data governance, transparency, diversity, non-discrimination, and fairness. These initiatives provide valuable resources for developers and policymakers working to mitigate algorithmic bias.
Future Directions
Regulatory and Policy ConsiderationsCurrent Landscape The regulatory landscape for AI is evolving, with several countries introducing laws to address AI ethics and bias. For instance, the European Union's AI Act proposes stringent requirements for high-risk AI systems, including mandatory bias mitigation measures. The act aims to ensure that AI systems used in critical areas like employment, law enforcement, and healthcare are transparent, traceable, and accountable.
Proposed Policies Proposed policies for AI governance often include mandatory bias audits, transparency requirements, and penalties for non-compliance. Such policies aim to create a legal framework that ensures AI systems are fair and accountable. For example, in the United States, the Algorithmic Accountability Act proposes that companies conduct impact assessments to identify and mitigate biases in automated decision systems. This policy aims to hold companies accountable for the fairness and transparency of their AI technologies.
Advancements in AI Research
Innovations in Bias Detection Research in AI continues to develop new methods for detecting and mitigating bias. Innovations such as explainable AI (XAI) help make AI systems more transparent, allowing developers to understand how decisions are made and identify potential biases. Techniques like SHAP (SHapley Additive exPlanations) and LIME (Local Interpretable Model-agnostic Explanations) provide insights into model predictions, helping to uncover and address biases.
AI for Social Good AI for social good initiatives focus on using AI to address societal challenges. These initiatives emphasize the importance of developing AI systems that benefit all members of society, particularly marginalized and underserved communities. For example, AI projects aimed at improving healthcare outcomes for underrepresented populations or using AI to enhance accessibility for people with disabilities demonstrate the potential for AI to contribute positively to society while ensuring fairness and inclusivity.
Conclusion
Moving Forward with AIImportance of Continued Vigilance: Addressing algorithmic bias is an ongoing process that requires continuous vigilance. Developers, policymakers, and stakeholders must work together to monitor AI systems and ensure they remain fair and unbiased. Regular reviews, updates to algorithms, and continuous learning from new data are essential to maintaining fairness in AI systems.
Collaboration Between Stakeholders: Effective mitigation of algorithmic bias involves collaboration between various stakeholders, including AI developers, ethicists, policymakers, and affected communities. By working together, these groups can develop and implement strategies that promote fairness in AI. For instance, multi-stakeholder initiatives like the Partnership on AI bring together diverse perspectives to address the ethical and societal implications of AI technologies.
To summarize, algorithmic bias in AI is a critical issue that affects various aspects of society. Understanding its sources, impacts, and mitigation strategies is essential for developing fair and ethical AI systems. Moving forward, continuous vigilance and collaboration among stakeholders are key to ensuring responsible AI development. We must collectively strive to create AI technologies that are transparent, accountable, and inclusive, promoting fairness and justice for all.
Should you have any queries or need further details, please contact us here.
Notification!