Custom AI Models and Local Deployment: A New Era for Enterprise Applications
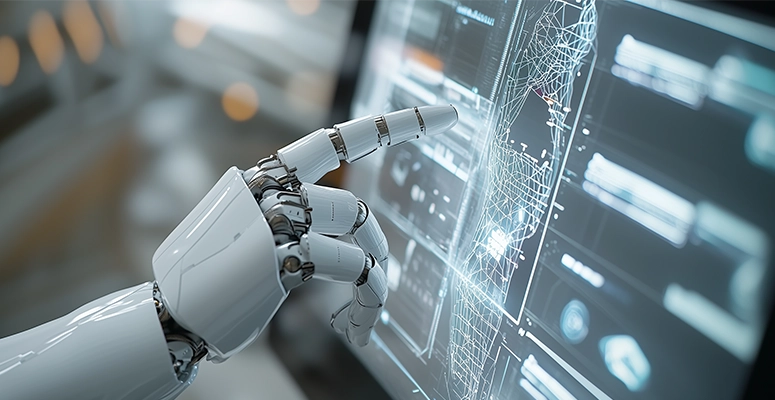
Artificial intelligence (AI) is transforming the way enterprises operate, providing new opportunities for efficiency and innovation. Custom AI models tailored to specific organizational needs are becoming increasingly popular. These models are designed to address unique challenges and leverage proprietary data to create highly specialized solutions. The evolution of AI has made it possible for enterprises to develop and deploy these custom models locally, ensuring that sensitive data remains secure and privacy concerns are addressed.
The significance of custom AI models lies in their ability to provide targeted solutions that generic AI models cannot offer. Historically, enterprises relied on off-the-shelf AI solutions that were not always a perfect fit for their specific requirements. The development of custom AI models marks a significant shift, enabling businesses to fine-tune algorithms and models to align precisely with their goals and data.
Drivers of Custom AI Model Development
Several factors are driving the development of custom AI models. One of the primary reasons is the need for specialized solutions that can address specific challenges within an organization. For example, in the healthcare sector, custom AI models can be designed to analyze patient data and provide personalized treatment recommendations. In finance, these models can be used for fraud detection and risk management, tailored to the specific patterns and anomalies present in an organization's data.
Enterprises often possess vast amounts of data that are unique to their operations. Custom AI models can leverage this data to create highly accurate and relevant solutions. Unlike generic models, which are trained on a broad dataset, custom models are trained on proprietary data, resulting in better performance and insights tailored to the organization's needs.
Privacy and security concerns also play a crucial role in the development of custom AI models. By deploying models locally, enterprises can ensure that sensitive data does not leave their premises, reducing the risk of data breaches and ensuring compliance with privacy regulations. This is particularly important in sectors like healthcare and finance, where data privacy is paramount.
The development of custom AI models has been greatly facilitated by the availability of robust open-source tools and frameworks. These tools provide a solid foundation for building, training, and deploying AI models without the need for significant upfront investment. Some of the most popular open-source AI frameworks include:
- TensorFlow: Developed by Google, TensorFlow is one of the most widely used AI frameworks. It supports a wide range of tasks, from simple linear regression to complex neural networks. TensorFlow's flexible architecture allows for easy deployment across various platforms, making it an ideal choice for custom AI model development.
- PyTorch: Developed by Facebook's AI Research lab, PyTorch is known for its dynamic computation graph and ease of use. It has gained popularity in both academia and industry for research and production applications. PyTorch's user-friendly interface and robust library support make it a preferred choice for developing custom AI models.
Using these open-source tools offers numerous benefits, including cost savings, flexibility, and community support. The collaborative nature of open-source projects means that developers can leverage the collective expertise of the community, access extensive documentation, and benefit from continuous improvements and updates.
Sector-Specific Applications
Custom AI models are particularly valuable in sectors that require specialized solutions and handle sensitive data. Let's explore how different industries are leveraging these models:
- Healthcare: In healthcare, custom AI models are being used to revolutionize patient care and medical research. For example, AI-driven diagnostic tools can analyze medical images with high accuracy, assisting doctors in the early detection of diseases like cancer. Personalized medicine is another area where custom AI models play a crucial role, analyzing patient data to recommend tailored treatment plans based on individual health profiles.
- Finance: The finance sector benefits significantly from custom AI models for tasks such as fraud detection, risk management, and investment analysis. AI models can identify unusual transaction patterns that may indicate fraudulent activity, enabling financial institutions to act swiftly and prevent losses. In risk management, custom models analyze vast amounts of data to predict market trends and assess the risk associated with various financial products, helping firms make informed decisions.
- Legal: In the legal industry, custom AI models are streamlining processes and improving efficiency. Document analysis is a critical application where AI can quickly sift through large volumes of legal documents, identifying relevant information and flagging potential issues. Predictive analytics is another valuable application, helping legal professionals anticipate case outcomes and develop more effective strategies.
These sector-specific applications highlight the versatility and impact of custom AI models. By addressing unique challenges and leveraging proprietary data, these models provide significant advantages over generic AI solutions.
Development Process of Custom AI Models
The development of custom AI models involves several key steps, each critical to ensuring the final model meets the organization's specific needs:
- 1. Identifying Specific Needs and Goals: The first step is to clearly define the objectives and requirements of the AI model. This involves understanding the problem to be solved, the desired outcomes, and any constraints or limitations. Engaging stakeholders from various departments helps ensure that the model aligns with the organization's broader goals.
- 2. Data Collection and Preprocessing: High-quality data is the backbone of any AI model. Collecting relevant data and preprocessing it to remove noise and inconsistencies is essential. This step may involve data cleaning, normalization, and augmentation to ensure the dataset is comprehensive and representative.
- 3. Model Selection and Customization: Based on the specific requirements, the next step is to select an appropriate AI model architecture. This may involve choosing from existing models or designing a new architecture. Customizing the model to fit the unique needs of the organization is crucial, as it ensures that the model performs optimally for the given task.
- 4. Training and Validation: Once the model is selected and customized, it needs to be trained on the preprocessed data. This involves feeding the data into the model, adjusting weights and parameters, and optimizing performance. Validation is performed using a separate dataset to ensure that the model generalizes well to new, unseen data.
- 5. Deployment and Monitoring: After successful training and validation, the model is deployed into the production environment. Continuous monitoring is essential to track the model's performance and identify any issues or drifts in accuracy. Regular updates and retraining may be necessary to maintain optimal performance as new data becomes available.
By following these steps, organizations can develop custom AI models that are tailored to their specific needs, ensuring high performance and reliability. Challenges and Solutions Developing and deploying custom AI models come with their own set of challenges. Addressing these challenges effectively is crucial for successful implementation. Here are some common challenges and their solutions:
- Data Privacy and Security: Handling sensitive and proprietary data poses significant privacy and security risks. Organizations must ensure that data is protected from breaches and misuse. Implementing robust encryption methods, access controls, and anonymization techniques can safeguard data. Additionally, adhering to regulatory requirements such as GDPR and HIPAA helps maintain compliance and trust.
- Integration with Existing Systems: Integrating new AI models with existing systems can be complex and time-consuming. Compatibility issues, data format mismatches, and system downtime are common hurdles. To overcome these, organizations should adopt modular and scalable AI solutions that can seamlessly integrate with their current infrastructure. Collaborating with IT departments and using standardized APIs can also facilitate smoother integration.
- Scalability and Performance: Ensuring that custom AI models perform well under varying loads and can scale efficiently is crucial. This requires robust infrastructure and efficient resource management. Leveraging cloud-based solutions and distributed computing can enhance scalability and performance. Regular performance testing and optimization help identify bottlenecks and improve the model's efficiency.
Addressing these challenges requires a combination of technical expertise, strategic planning, and ongoing monitoring to ensure that custom AI models deliver the desired results.
Ethical Considerations
As custom AI models become more prevalent, ethical considerations play a vital role in their development and deployment. Ensuring that AI systems are fair, transparent, and compliant with regulations is essential to maintaining public trust and avoiding unintended consequences.
- 1. Ensuring Fairness and Avoiding Bias: AI models can inadvertently learn and perpetuate biases present in training data. This can lead to unfair outcomes, particularly in sectors like healthcare and finance. To mitigate this, organizations should implement techniques such as bias detection and correction, diverse training datasets, and regular audits to ensure fairness in AI outcomes.
- 2. Transparency and Explainability: Complex AI models, particularly deep learning models, can often act as "black boxes," making it difficult to understand their decision-making processes. Ensuring transparency and explainability helps build trust and allows stakeholders to comprehend how AI models arrive at their conclusions. Techniques like model interpretability tools and providing clear documentation can enhance transparency.
- 3. Compliance with Regulations: Adhering to legal and regulatory requirements is crucial for the ethical deployment of AI models. Organizations must stay updated with evolving regulations and ensure their AI systems comply with standards like GDPR, HIPAA, and other relevant laws. Regular compliance checks and legal consultations can help maintain adherence to these regulations.
By addressing these ethical considerations, organizations can develop and deploy custom AI models responsibly, ensuring they contribute positively to their respective sectors and society at large.
Future Trends
The development and deployment of custom AI models are poised to evolve significantly, driven by ongoing advancements in technology and growing adoption across various sectors. Here are some future trends that are likely to shape this landscape:
- 1. Advances in AI Technology: Emerging technologies such as quantum computing, neuromorphic engineering, and advanced machine learning algorithms will further enhance the capabilities of custom AI models. These advancements will enable more complex and accurate models, opening up new possibilities for solving intricate problems and improving decision-making processes.
- 2. Increasing Adoption in New Sectors: While sectors like healthcare, finance, and legal are currently leading the way, other industries such as manufacturing, retail, and agriculture are expected to increasingly adopt custom AI models. For instance, AI-driven automation and predictive maintenance in manufacturing can enhance productivity and reduce downtime. In retail, personalized marketing and inventory optimization can be achieved through custom AI solutions.
- 3. Evolution of AI Governance and Best Practices: As the use of custom AI models becomes more widespread, the establishment of governance frameworks and best practices will be crucial. Organizations will need to develop comprehensive AI strategies that include ethical guidelines, risk management, and continuous improvement processes. Industry standards and regulatory bodies will play a key role in shaping these frameworks.
These trends indicate a promising future for custom AI models, with the potential to drive significant innovation and value across various sectors.
Conclusion
The rise of custom AI models marks a significant milestone in the evolution of artificial intelligence within enterprises. By leveraging open-source tools and frameworks, organizations can develop specialized AI solutions tailored to their unique needs. This approach not only enhances efficiency and accuracy but also ensures data privacy and security, which are paramount in sectors such as healthcare, finance, and legal.
The development process of custom AI models involves careful planning, data preprocessing, model selection, training, and deployment. Overcoming challenges related to data privacy, system integration, and scalability requires a combination of technical expertise and strategic planning. Ethical considerations, including fairness, transparency, and regulatory compliance, are essential to ensure responsible AI deployment.
Looking ahead, advancements in AI technology, increased adoption across new sectors, and the evolution of governance frameworks will further shape the landscape of custom AI models. As organizations continue to innovate and refine their AI strategies, the impact of custom AI models on enterprise applications will only grow, driving significant improvements in performance, efficiency, and decision-making.
Should you have any queries or need further details, please contact us here.
Notification!